Deep Learning on Graphs for Natural Language Processing WWW 2022
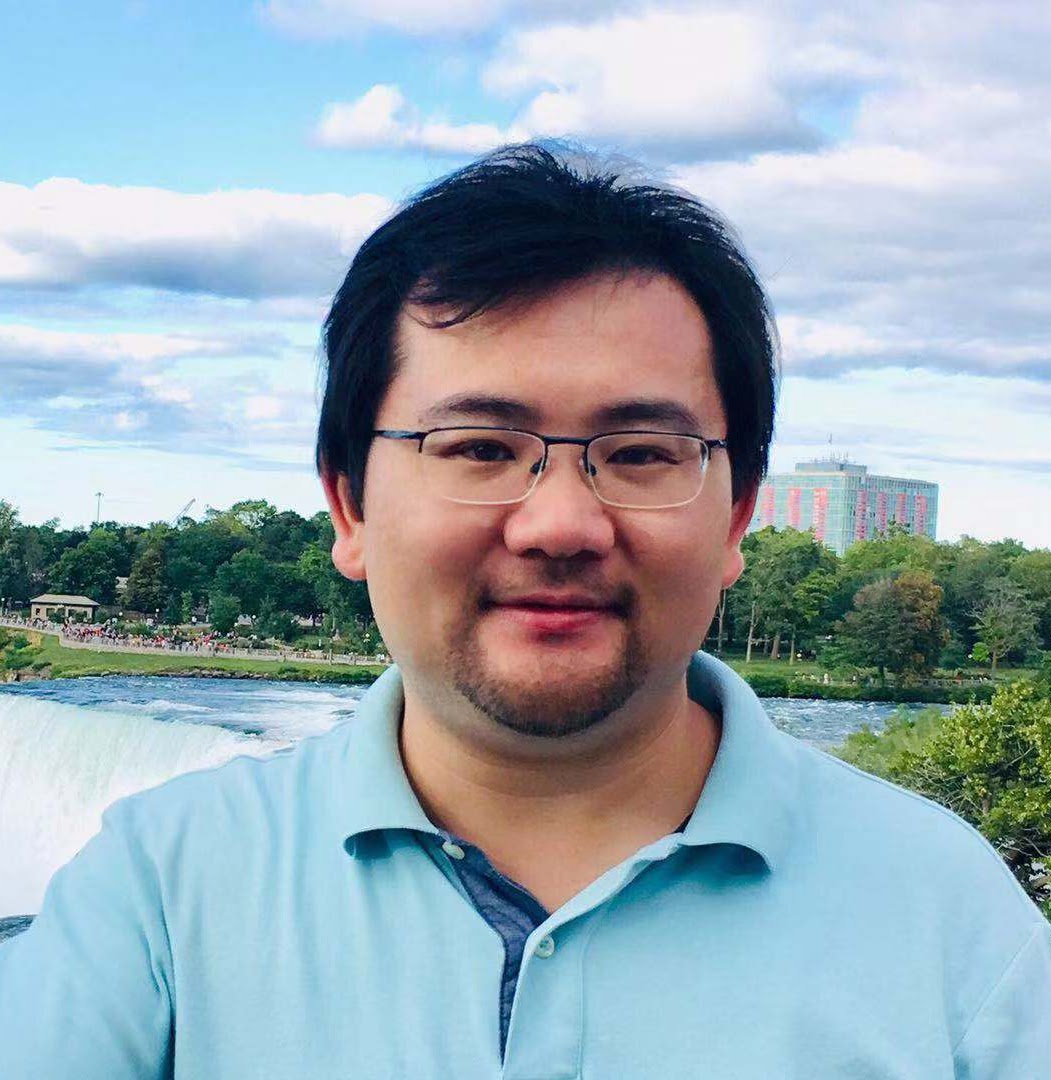
Lingfei Wu, JD.COM Silicon Valley Research Center
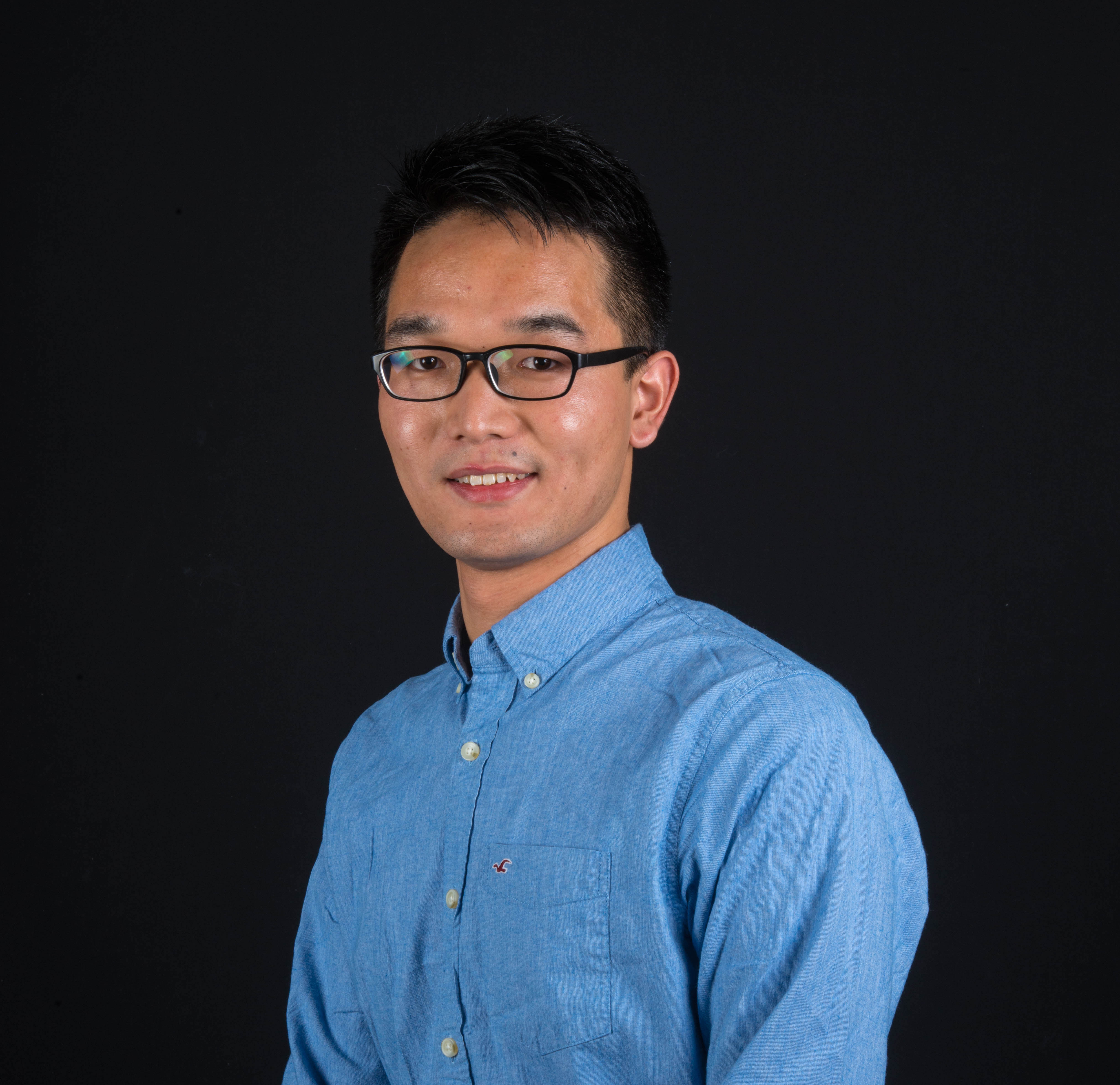
There are a rich variety of NLP problems that can be best expressed with graph structures. Due to the great power in modeling non- Euclidean data like graphs, deep learning on graphs techniques (i.e., Graph Neural Networks (GNNs)) have opened a new door to solving challenging graph-related NLP problems, and have already achieved great success. Despite the success, deep learning on graphs for NLP (DLG4NLP) still faces many challenges (e.g., automatic graph construction, graph representation learning for complex graphs, learning mapping between complex data structures).
This tutorial will cover relevant and interesting topics on apply- ing deep learning on graph techniques to NLP, including automatic graph construction for NLP, graph representation learning for NLP, advanced GNN based models (e.g., graph2seq and graph2tree) for NLP, and the applications of GNNs in various NLP tasks (e.g., ma- chine translation, natural language generation, information extrac- tion and semantic parsing). In addition, hands-on demonstration sessions will be included to help the audience gain practical experi- ence on applying GNNs to solve challenging NLP problems using our recently developed open source library – Graph4NLP, the first library for researchers and practitioners for easy use of GNNs for various NLP tasks.